The 133rd episode of Datacast is my conversation with Salma Bakouk, who is the CEO and co-founder of Sifflet, a Full Data Stack Observability platform.
Our wide-ranging conversation touches on her love for mathematics growing up, her early career working in investment banking, the founding story of Sifflet the concept of full data stack observability, Sifflet’s approach to data quality/lineage/catalog, building a modern data team, lessons learned from hiring and building a startup culture, and much more.
Please enjoy my conversation with Salma!
Show Notes
(01:41) Salma reflected on her upbringing in Paris (France) and her love for math since a young age.
(04:05) Salma talked about her decision to dive deeper into statistics.
(07:39) Salma recalled her 5 years in investment banking in Hong Kong.
(10:44) Salma doubled down on the cultivation of her resilience during this period.
(13:45) Salma shared the founding story Sifflet.
(15:53) Salma touched on her co-founder dynamics with Wajdi Fathallah and Wissem Fathallah.
(18:50) Salma explained the concept of Full Data Stack Observability to the uninitiated.
(22:14) Salma extrapolated on Sifflet's approach to data observability.
(24:37) Salma discussed Sifflet's data quality focus with automated monitoring coverage and over 50 data quality templates.
(27:28) Salma discussed Sifflet's data lineage solution with field-level lineage, root cause analysis, and incident management/business impact assessment.
(31:47) Salma discussed Sifflet's data catalog with a powerful metadata search engine and centralized documentation for all data assets.
(33:46) Salma expanded on the full-stack mindset as a data vendor.
(37:03) Salma emphasized the importance of integrations with other data tools.
(38:52) Salma touched on product features such as Flow Stopper to stop vulnerable pipelines from running at the orchestration layer and Metrics Observability to extend the observability framework to the semantic layer.
(43:40) Salma unpacked her article on building a modern data team.
(47:54) Salma shared some hiring lessons to attract the right people to Sifflet.
(54:02) Salma emphasized the importance of company branding.
(55:01) Salma shared her thoughts on building a startup culture.
(57:26) Salma briefly mentioned her process of working with design partners in the early stage.
(01:00:17) Salma emphasized her enthusiasm for the broader data community.
(01:02:05) Conclusion.
Salma's Contact Info
Sifflet's Resources
Mentioned Content
People
Zhamak Dehghani (Creator of Data Mesh and Founder of NextData)
Benoit Dageville, Thierry Cruanes, and Marcin Zukowski (Founders of Snowflake)
Books
"The Hard Thing About Hard Things" (by Ben Horowitz)
"The Boys In The Boat" (by Daniel James Brown)
Notes
My conversation with Salma was recorded back in late 2022. Since then, I recommend checking out the launch of Sifflet AI Assistant and this blog post on 2024 data trends.
About the show
Datacast features long-form, in-depth conversations with practitioners and researchers in the data community to walk through their professional journeys and unpack the lessons learned along the way. I invite guests coming from a wide range of career paths — from scientists and analysts to founders and investors — to analyze the case for using data in the real world and extract their mental models (“the WHY and the HOW”) behind their pursuits. Hopefully, these conversations can serve as valuable tools for early-stage data professionals as they navigate their own careers in the exciting data universe.
Datacast is produced and edited by James Le. For inquiries about sponsoring the podcast, email khanhle.1013@gmail.com.
Subscribe by searching for Datacast wherever you get podcasts, or click one of the links below:
If you’re new, see the podcast homepage for the most recent episodes to listen to, or browse the full guest list.
Key Takeaways
Here are the highlights from my conversation with Salma:
On Her Love For Math Growing Up
I was born in Morocco and grew up in Paris, France. I have a degree in applied math statistics and an engineering degree in applied math.
Growing up in Paris was exciting. It is a lovely city, rich and increasingly international. In high school, I focused solely on math and wasn't interested in other subjects. I was put on a particular math track involving intense maths and physics study.
Afterwards, I took an exam, and based on my national ranking, I had the opportunity to attend Ecole Centrale Paris, one of the prestigious engineering schools in Paris. I continued studying math and earned a degree in applied math.
On Diving Deeper Into Statistics
It was during my time at engineering school that I pursued my degree. My major was applied mathematics, and I chose a minor in contemporary art, which was quite random.
In my final year, I had the opportunity to specialize in a specific area, so I decided to focus on statistics. I pursued a double degree with Universite Paris Dauphine - PSL and obtained a second master's degree in statistics. It was a challenging experience as I was simultaneously taking both courses.
Despite the intensity, I thoroughly enjoyed it and found that it laid a strong foundation for building resilience in my future career. At the time, data science was emerging as a field of interest, and I was particularly passionate about statistics. While mathematics can be quite theoretical and abstract, I became increasingly drawn toward finding practical applications for the theories I was learning.
One project that stands out in my memory was during a statistics course. We were tasked with finding a real-life application for the concepts we studied. I had the opportunity to collaborate with a large gaming company to develop a predictive model for analyzing player behavior and creating game challenges based on that information. What made this project even more fascinating was the involvement of psychologists who provided insights into human behavior and psychology.
Working alongside them, we aimed to translate their expertise into something that could be incorporated into the predictive model. It was a unique experience that resonated with me over the years, as it combined advanced statistical principles and mathematics with a multidisciplinary approach.
Interestingly, I could have pursued psychology in another life as I have a deep passion for the subject. This project reinforced my interest in psychology and its potential applications. If given the chance, I would choose to study psychology again.
It's intriguing how my path led me to the field of data, which aligns with your observations. Data science requires a solid technical foundation and leverages business principles to drive value within organizations. It encompasses both the technical aspects and the human interactions that contribute to the success of businesses. This combination is what I find most enjoyable in my work.
On Being An Investment Banker
My experience in investment banking was intense and incredible. I learned a lot during that time. Right after graduating from college in France, I was offered a job in Hong Kong. Since I had never been to Hong Kong, it was a complete adventure. I ended up moving there in 2015.
I joined one of the largest US banks as an analyst in the equity center trading division. I was quickly immersed in an environment where real-time data was used to make significant decisions. It was an intense and high-stress environment, which involved managing large sums of money daily.
I thrived in that environment and discovered aspects of my personality that I didn't know existed. It was a fantastic learning experience, and I had the opportunity to work with incredibly intelligent individuals. Specifically, regarding data initiatives, we used real-time data in the equity trading division to make decisions and provide trade recommendations to clients and the bank. Any delay or problem with the data we received was a major issue.
As the expectations for data delivery were high from both internal and external stakeholders, I was responsible for ensuring the quality of the data. Despite working for a bank with a modern infrastructure and highly skilled technical staff, access to data was limited to a select group of individuals. There was no data democratization, which made it challenging for the business to become data-driven.
To address this issue, I led initiatives to democratize data and improve access for both business stakeholders and other data consumers. We invested significant time and resources in creating an intelligence layer. However, we still encountered data quality issues even with a modern data stack. This became a major obstacle in adopting a data-driven culture.
These challenges personally motivated me and led to the creation of Sifflet.
On Cultivating Resilience
The bank I worked for was well-known for its strong culture, which was one of the best things about working there. Starting my career in such an environment instilled strong values in me and shaped my perspective on goals, achievements, and ambition.
The company had a culture centered around winning, excellence, and ambition. Being surrounded by highly intelligent colleagues constantly challenged me and motivated me to push my potential as part of a team.
Working in that environment helped me develop resilience, especially in the unique setting of the trading floor. Resilience became stronger through exposure to high expectations, potential conflicts, and tense encounters.
The experience at the bank continues to benefit me as a founder and will stay with me for life. It taught me valuable lessons that I apply in my career as an entrepreneur. The environment where I started my career played a significant role in shaping the person I am today.
In summary, working in that environment helped me build resilience and grit. It provided me with a playbook on how to deal with various situations, learn from positive and negative experiences, and adapt quickly.
On The Founding Story of Sifflet
I met my co-founder in engineering school 10 years ago, and we've been best friends ever since. Our initial bond was formed over math models.
Over the years, we stayed in touch while Wissem pursued his career at Uber, Amazon, and then Uber again. Wadji, who shares the same last name, is his older brother. That's how the three of us know each other. While working in investment banking, I reached out to both of them for advice and to learn from their experiences as data practitioners at tech-savvy companies. I wanted to apply their best practices to my own job.
This conversation started around 2017-2018. When we began encountering data quality issues at my company, I asked them for help. Surprisingly, they were facing similar challenges in their respective companies. We realized that these issues were causing problems for many companies of different sizes and industries.
After extensive research, we decided to leave our jobs and start our own company in January 2021. And that's the story.
On The Co-Founder Dynamics
Because we have known each other for a long time and have been close friends, I know that we share the same values and have similar views on how the world works. This is extremely important in a co-founder because you need to be ambitious when you start a company. Both co-founders must have the same level of ambition, hunger, and excitement about building and creating things.
Resilience and grit are also essential traits. I know them personally, and I am aware of the experiences they have been through and what they have overcome. They are very resilient people. This is important because when starting a company with co-founders, ideally, your down moments coincide with their moments of optimism, balancing things out. I could never be a solo founder, but I have a high level of respect for solo founders. In my case, I can vent and express my exhaustion or doubts because of our personal relationship. We can have those conversations without questioning each other's commitment to what we are building together.
Another critical point is that knowing and trusting each other becomes a superpower for the company's execution. As the CTO, CPO, and CEO, we have distinct roles and complementary personalities. When Wadji makes a technology decision, I don't need him to explain it, even though I have the background to understand. The same goes for Wissem and vice versa. This efficiency in decision-making is helpful in a co-founder relationship.
On Full Data Stack Observability
Observability is a concept derived from software engineering. In software engineering, observability relies on three main pillars: metrics, traces, and logs. Over the past decade, companies like Datadog, New Relic, and Splunk have emerged to address the problem of lack of visibility and help modern organizations achieve observability. These companies provide a comprehensive view of the infrastructure and applications, allowing software engineers to monitor the health status and ensure sustainable functioning.
The idea behind software observability is to provide software engineers with detailed information about the functioning of their applications and the infrastructure they run on. This enables them to monitor the health status and ensure smooth operation. For example, streaming services like Netflix rely on software observability to ensure uninterrupted service.
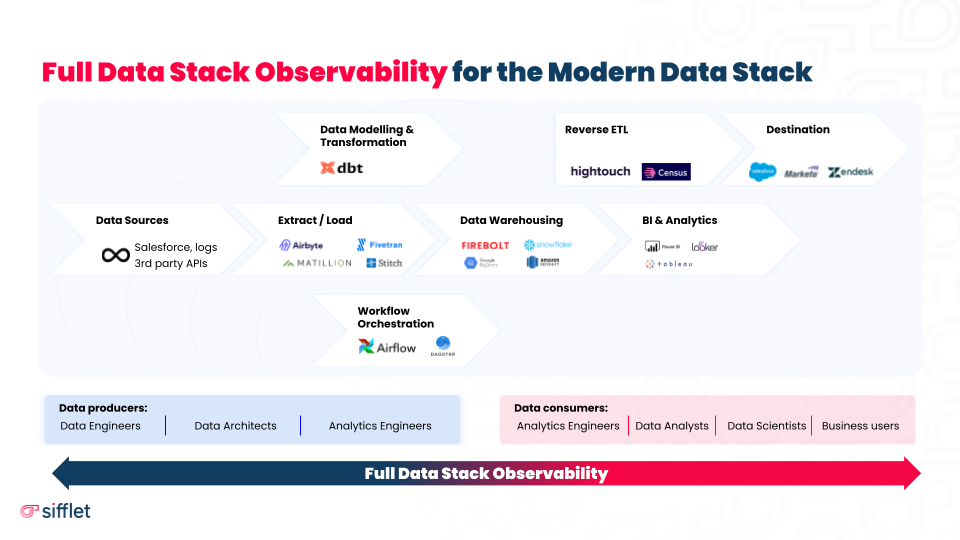
Data observability is a relatively new category that aims to provide a similar bird's eye view and full visibility into data assets. It helps companies establish trust and reliability in their data by collecting and analyzing signals across the data, metadata, and infrastructure. Data observability is based on three pillars: metrics, metadata, and lineage.
Metrics involve data quality rules and controls that define the health status of data assets. This includes freshness, completeness, distribution, volume, and schema changes.
Data observability looks for anomalies at the data level, such as receiving an email address instead of a zip code or receiving a zip code that doesn't match the expected format.
Additionally, the infrastructure supporting the data product, including pipelines, must properly function to ensure data integrity and prevent business catastrophes.
Overall, the goal of observability, whether in software or data, is to provide a holistic view and enable proactive detection of anomalies for efficient operation.
On Sifflet's Approach
Data quality issues have been present as long as businesses have used data to leverage their operations. This is not a new problem. However, in recent years, there has been a significant revolution in the field of data engineering and data infrastructure, which has made data integration processes much easier and automated. This has also allowed for the realization of many use cases.
Today, companies are embracing complexity and relying on various external data sources. They want to ensure that their investment in data infrastructure provides a good return and that everything is functioning properly. This includes ensuring that business users have access to reliable data. As a result, data quality monitoring and management projects are gaining more attention.
Observability, a concept borrowed from other industries, now plays a crucial role in the context of the modern data stack. Many solutions to this problem are emerging under the new framework of data observability.
Sifflet takes a full-stack approach as a top-of-stack layer that sits on an existing data stack. We offer anomaly detection, root cause analysis, incident management reporting, and monitoring and observability for data assets at any point in the value chain.
On Data Quality
Our data quality monitoring and anomaly detection approach is based on two approaches. First, we have a purely machine learning-based approach. I am particularly passionate about this topic because ML-based anomaly detection should either be done right or not at all.
The second approach we use for data quality monitoring and anomaly detection is rule-based. This allows us to cover more niche use cases and will enable users to define their own criteria. While we have our own set of quality metrics based on our experience, we recognize that users know their use cases best and what they want to monitor.
In terms of metrics, we look at data anomaly detection from three perspectives. First, we examine data anomalies at the data level, such as drift within the data, format validation, and field profiling. For example, we ensure that a zip code is in Paris when it should be.
Second, we consider metrics related to freshness, volume, and changes in schema. These metrics help us identify any deviations in the data and ensure its accuracy.
Lastly, we go beyond data and metadata anomalies and examine anomalies at the infrastructure layer. This allows us to be even more proactive in addressing data quality issues. We can prevent issues from propagating downstream by detecting anomalies in the infrastructure layer. This is important because a broken pipeline consumes resources and can lead to data issues. We aim to detect anomalies while the pipeline is running so that we can take immediate action and troubleshoot effectively.
To ensure proper functioning at the pipeline level, we have connectors that integrate with other tools such as dbt, Airflow, and Firebolt. These connectors help us monitor and address issues before they become data and metadata anomalies.
This comprehensive approach sets us apart and ensures high data quality.
On Data Lineage
First of all, lineage is a concept that is still somewhat misunderstood, and there is still a lot of confusion around it. When we sat down to think about what we wanted the tool to achieve, there were differences in background and perspective between my co-founders and me. I come from a data consumer and business leader background, whereas my co-founders come from a pure analytic and data engineering background.
During our discussions, we took on the roles of a data team, each representing a different persona. This mirrors the uncertainty around ownership of data quality that we see with our customers. Often, people take ownership once someone at the C level becomes frustrated with receiving inaccurate numbers. Then, people start to think about and address data quality. This is the problem we want to solve - data entropy.
This is where the full data stack approach comes into play. We wanted a tool that could cater to data engineers and consumers, helping them become more proactive about data quality management. However, these two personas are quite different and struggle to communicate effectively with each other. Our challenge was to create the "glue" between them, which is the lineage.
In fact, the very first lines of code we wrote were to build a lineage solution. We wanted to create that connection between data engineers and data consumers and provide context to the anomalies detected by the tool. When you receive an alert about a rule failure or a breaking issue, knowing what to do with that alert is crucial. Many data quality initiatives and troubleshooting efforts fail because it is impossible to manually track the relationships between data assets in a modern and complex infrastructure.
By leveraging lineage, one of our product's pillars, we can provide upstream context for root cause analysis to data engineers and downstream impact analysis to data consumers. This is what we refer to as incident management and business impact assessment. It allows both personas to receive actionable information and insights, helping them proactively manage data issues.
This explains how we leverage lineage and how Sifflet's approach has benefited customers. Troubleshooting data incidents is often the most challenging part, consuming roughly 50% of data engineers' time. With our end-to-end lineage, we can optimize our data teams' resources by automatically computing the lineage and pinpointing the root cause. At the same time, we inform data consumers about potential impacts. This is why lineage is a compelling and key feature of our product.
On Data Catalog
We published a blog post highlighting our philosophy behind having a catalog in our product. Data teams or business teams often use data catalogs as a starting point to discover and find the assets they need to use.
However, if you can't trust the data you find in your data catalog, then having a data catalog becomes pointless because you'll just be searching for low-quality data. That's why we prioritize data quality and health information, and the best way to present it to the user is through a catalog.
Think of the catalog as the entry point for lineage. You search for your data assets in the catalog, and importantly, you can see the health status, lineage, and the teams using those assets. We have a feature called "temperature" that indicates the quality of the assets and more. This helps you get closer to a single source of truth.
Having data catalog and data observability within the same tool or platform makes a lot of sense in achieving this single source of truth everyone talks about.
On The Full-Stack Mindset
Our approach with Sifflet is to pioneer the full data stack observability platform. This approach allows us to fit on top of any existing data stack, regardless of whether it is highly fragmented, decentralized, or relies on various data sources. With full data stack observability, you can navigate through the data, make sense of it, and reduce what we refer to as data entropy.
Companies are realizing that complexity and data entropy are inevitable when they are growing and expanding. Our goal with Sifflet is to enable companies to maximize the value of their data assets and embrace the flexibility that comes with using multiple tools and data sources.
To achieve this, it is crucial to ensure that the output of the data platform is reliable and trustworthy. This is where data plays a vital role. The modern data stack is an evolving ecosystem, and it is exciting to be building a company in this space, similar to my experience with data at Goldman Sachs.
Data observability, particularly the Sifflet approach, focuses on being full stack. We don't concern ourselves with how complex or fragmented the data stack becomes because we act as the glue that ensures everything works properly, is reliable, and is visible.
Monitoring and observability are essential, but if you only focus on one area of the data stack, you may excel in that specific aspect. For example, you may have a powerful anomaly detection model that works perfectly with the warehouse. However, what happens before the data enters the warehouse or after it leaves? It is crucial to address potential issues in these areas as well.
In our opinion, the full stack approach is the only way to embrace the complexity of modern infrastructure and optimize the return on investment in your data infrastructure, whether that involves people, technology, or other resources.
On Integrations
We anticipated the need for integrations early on, so we built a lean microservice infrastructure. This infrastructure makes us highly agile in creating integrations and shipping new features. We knew that integration would be a major focus for us, so we dedicated a team to handle integrations.
When prioritizing integrations, we consider factors such as go-to-market strategies, customer satisfaction, and industry trends. We strive to make decisions that make sense for our company and customers.
On Flow Stopper
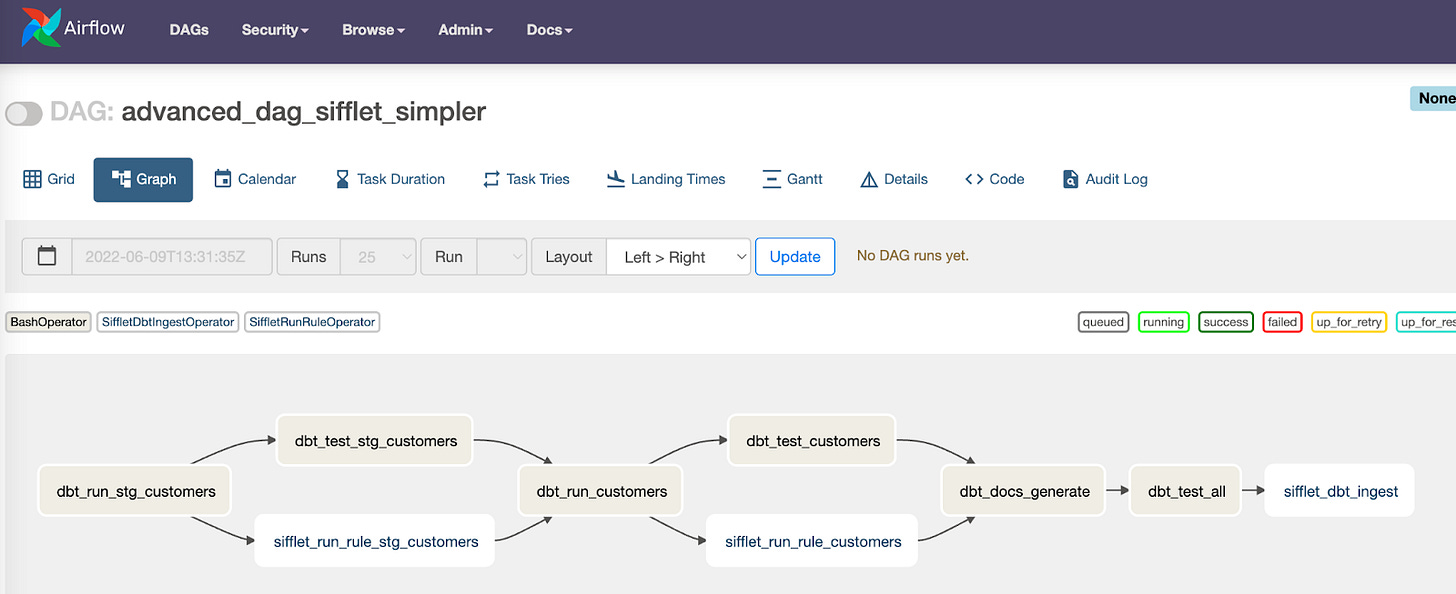
The Flow Stopper feature addresses the need to detect data quality issues at the pipeline level before they impact data in production. To achieve this, we connect to the orchestration layer.
Initially, we developed a native connector for Airflow, but it proved insufficient. That's when the Flow Stopper feature came into play. Flow Stopper lets you connect your chosen arbitrator, whether Airflow Vector or any other tool.
We achieve this connection either through a native integration like Airflow Vector or via the command-line interface (CLI). At the pipeline level, we insert a data quality rule or quality control mechanism to ensure that anomalies can be detected at the orchestration layer. In other words, if an anomaly is identified, data engineers are alerted to potential pipeline issues and can stop it to troubleshoot before it further impacts the business.
Flow Stopper enables data engineers to integrate testing into their pipeline at the orchestration layer. This ensures continuous monitoring, prevents broken pipelines from consuming resources, and maintains data integrity.
On Metrics Observability
The metrics layer serves as a translator between data, the warehouse, and the business. This is an excellent step towards data utilization.
However, non-technical users, specifically business users, may feel uncomfortable using data assets without knowing their source, how they are computed, and their reliability. As part of our full data stack observability framework, we can track data lineage from ingestion to consumption and push that lineage to the semantic layer.
Our catalog allows users to create or import metrics from a metric store, a BI tool, or directly from dbt. This will enable users to discover metrics and access information such as data quality rules, health status, lineage, usage, etc. By expanding the boundaries of lineage, we empower business users to have greater confidence in the data assets they use.
This feature reflects our commitment to enabling self-serve analytics rather than just being a buzzword. We are witnessing a growing focus on the semantic layer, and observability and reliability are becoming important topics. After all, data cannot be effectively provided to consumers without a certain level of confidence in its reliability.
On Building A Modern Data Team
The article is primarily aimed at two groups: those new to building a data practice and those who come from different backgrounds but are working on data-related projects involving various internal stakeholders, such as engineering and business.
I can personally relate to this position as well. In the past, I was a business leader who had to transition my team to become data-driven. Since I was their leader, it was my responsibility to take the initiative in making the team data-driven.
Although I have a theoretical engineering background and some coding knowledge, I felt overwhelmed when assigned this intelligence layer. I had no idea where to start and felt intimidated by the rapidly evolving technology space.
I hesitated to reach out to my friends in the data field and ask questions like, "What is dbt?" or "How can I create a dashboard from our database?" I also had other concerns, such as how to hire the right people for the data team and what the data platform should look like.
I spent a lot of time researching and learning from the people around me, but I made mistakes along the way. The purpose of this blog is to reflect on my experience and guide those going through a similar situation. It serves as a playbook for those who feel lost and need a starting point.
The blog covers many topics, from basic ones like data team architecture and use cases to more advanced considerations such as whether to adopt a centralized or data mesh approach and what tools to look for. It also addresses the hiring aspect, discussing whether the first hires for the data team should be full-stack with SQL fluency or possess software engineering skills.
I provide examples and insights based on the level of maturity and expectations of the business. It's important to note that a company in its early stages will have different data expectations than an established one.
This is the essence of the blog and why we published it - to share knowledge and help others navigate the challenges of building a data practice.
On Hiring
I did a lot of hiring in the past when I was in my previous role. But at the time, attracting candidates was actually relatively easy. I would simply say, "Hey, I'm hiring for the best, the largest bank in the world. Do you want to come join us?" So, the brand was helpful. When we started hiring at Sifflet, we faced some challenges, especially in the early days when my co-founders and I were looking to make our first hire. We didn't have that brand yet.
We couldn't even clearly articulate the product's vision, so we really had nothing to say. So, we decided to look within our network to find people we knew. The first hire after the co-founders is almost like hiring another founder. They become an extension of the founding team.
We were incredibly lucky because we hired someone we had known for a decade. We went to school together and knew what to expect regarding their personality fit. We also aligned on values such as resilience, ambition, and grit. So, regarding technical abilities, it was just a matter of checking the boxes. This was the easiest part.
From there, we continued to leverage our network. For example, our first hire, Al, brought along someone from his previous team, and my co-founder hired some people from his previous team as well. At this point, we had 10 employees, and we all knew each other and had worked together in the past.
Now, the challenge was expanding beyond our comfort zone of knowing everyone and bringing in new people while also selling the company as a brand to new hires. This involved a lot of trial and error, but improving our ability to sell the company and its vision was important. We started to have proof points like early customers and investors, which we could show to future hires to demonstrate the opportunity and get them excited about it.
I learned the hard way in my previous experience that when you hire extremely smart people, you need to give them space and opportunity to grow. You can't hire someone who's extremely smart and tell them what to do. As a founder, it's a fine line to walk. You need to acknowledge their expertise and ask for their input.
During interviews, we now give candidates a product demo and ask them to point out one or two things we're doing wrong or poorly. We want to know what they would change if they joined us. This not only gives candidates the confidence to explore their full potential and bring value to the company, but it also assures them that they are not joining a clique where everyone has known each other for years.
As the team grows, we work on building a sense of culture and belonging. We communicate on everything and anything. We have an office, but many people are remote, so we try to meet up regularly. We have a Monday meeting every week at 2:00 PM to discuss various topics, except work. We talk about personal matters like how people are doing, who's getting married, who's getting a dog, who went on a hike, who's sick, who's burnt out, and so on. This helps create a sense of community and deepens our understanding of each other on a personal level. It also contributes to our company culture as we learn how people react in different situations.
I feel extremely fortunate to work with such amazing people on our team. We are growing rapidly, so if anyone is interested, please contact me.
On Branding
Branding is of utmost importance, even in the early stages of a business. It is crucial to be mindful of how you are perceived externally. This not only impacts customers and overall visibility but also plays a significant role in hiring.
For instance, just recently, I tweeted about this. Someone read one of our blog articles and applied for a position. During the interview, he mentioned that he applied because he had read the blog and believed we were building a great product. This highlights the impact of small improvements in overall branding, which can make the company more appealing as a potential workplace.
I have consistently emphasized to our marketing team the need for a frequently asked questions (FAQ) page on our website that explains what Sifflet is. In French, Sifflet means "Whistle," which represents the alerting aspect of our product.
On Defining Company Culture
So, when we knew we would start hiring people, we sat down with my co-founders and tried to write down our company values. We wanted these values to form the foundation of our company culture. However, we didn't communicate this to anyone and kept it between us. We decided to revisit this topic a year later. We sent an email to reopen the discussion and see if those values still hold true today.
A year later, I went on the company Slack and shared the list of values. I asked everybody to vote on what they believe should be the company's values. Through this process, we developed an interesting set of values that align with our team's growth. We realized that values cannot be simply defined by default. Values should be inherent, don't require much thought, and come naturally.
Instead of imposing our values on the team and expecting them to respect and communicate them, we felt it was better to create and define them together as a team. We plan to repeat this exercise a year from now when we have a larger team. I will ask everyone to vote on what they think the company's values should be. This exercise is an ever-evolving process and shouldn't be solely decided by the founders or leadership.
The values we establish are a building block of our company's culture and how people interact with each other. It should be a collective effort from everyone.
On Working With Design Partners
So, in the early days, and even at our current stage, our customers are early adopters because our company is still relatively young. In the beginning, we relied on our network and the networks of our investors and advisors to find companies that faced similar challenges to the ones we were trying to solve.
While every company has data quality issues, not every company experiences them at the same level. We wanted to work with companies that not only had these challenges but also had the willingness to collaborate, provide feedback, and iterate with us. Additionally, it was important to us to work with decent human beings who we enjoyed partnering with, as this is a partnership.
We consider our clients design partners, so the relationship must be mutually beneficial. This approach allowed us to work with some of the most amazing companies in the world from the start of our journey, and we feel fortunate to have had them join us early on. When working with design partners, it is crucial to stay true to the DNA of your product and have a clear idea of your mission, at least as a founder or founding team.
While gathering inputs and feedback from design partners, as they use the tool and are data practitioners, it is equally important not to rely solely on their guidance. Some companies start from scratch and hope that design partners will dictate the direction of the company or the product. This is not the ideal approach for building a company or a product.
As a founder, having experience in the industry or problem space you are trying to solve is beneficial. This lets you know how you envision the tool or the approach to solving the problem. Engaging in discussions with a broader community of design partners and seeking feedback from external sources can help validate your ideas and guide you in the right direction.
On The Data Community
Honestly, at the beginning, I was pleasantly surprised by how open and friendly the data and analytics community is. When I started thinking about building intelligence at my previous company, I felt extremely intimidated.
I was scared to reach out to others within my organization and ask them questions I thought might be silly. However, now that I'm in this space, I've realized that people love to talk about their experiences. There are numerous communities on Slack, meetups, and other events. It has honestly been the most surprising aspect for me.
It has also been a valuable resource. Being connected to a vendor in this field is like having a super boost. If you have any questions or are struggling with something, you can reach out to someone on LinkedIn or Twitter and find someone who can provide answers.
This is what I find remarkable about the data community. I am extremely fascinated by the mindset of its members and feel incredibly lucky to be building my career in this field.
Share this post